AI-powered job matching: Connect with decision makers and land your dream job in tech effortlessly (Get started for free)
7 Data-Driven Strategies to Transform Biased Performance Reviews into Objective Assessments
7 Data-Driven Strategies to Transform Biased Performance Reviews into Objective Assessments - Implementing Standardized Metrics Through Performance Data Dashboards
As we move into late 2024, there's growing recognition of the need to overhaul performance review processes. The key to transforming subjective and potentially biased performance reviews into objective evaluations is to integrate measurable, standardized metrics through accessible performance data dashboards. These dashboards bring a sense of order to the chaotic world of employee assessment by laying out performance indicators in a way that's easy to understand. They don't just look nice; they're designed to keep individual evaluations directly connected to the larger objectives an organization is striving to achieve. But let's be clear: implementing these dashboards isn't just a technical exercise. It demands a well-thought-out approach to how data is gathered, ensuring that the information being fed into these systems is both trustworthy and gathered in a consistent manner. And even with the best data, there's the lingering threat of bias creeping in during analysis, a challenge that must be actively managed. If done right, these dashboards do more than just track numbers. They facilitate a dynamic discussion among stakeholders, creating a framework where performance is evaluated transparently and improvements can be identified collaboratively. In essence, this push towards data-driven performance reviews is about creating a work environment where evaluations are rooted in fairness, encouraging a shift toward a more equitable assessment experience for everyone involved.
Utilizing performance data dashboards to roll out standardized metrics might sound straightforward, but it's a multifaceted endeavor. We have to start with the basics, what are we even measuring and why? The alignment of metrics with wider organizational goals is paramount, ensuring that we're not just collecting data for the sake of it but to derive meaningful insights. Does that even work in the real world and what are its downsides? You would think this is a given, but my research suggests that this often gets overlooked. Also, I wonder how practical these dashboards are. It would be interesting to gather more data on that.
A holistic view of employee performance necessitates incorporating diverse metrics. It's not just about output, but also how one reaches that output. For instance, are quarterly reviews, productivity rates, and attendance records truly indicative of someone's value to an organization? How often are these dashboards updated, and what data is needed? And who interprets this data? If the data is sound, who ensures a human element is maintained when evaluating a person?
The challenge of creating bias-free measures is a tough nut to crack. It seems obvious that accurate interpretation and the actual use of data in assessments are critical. However, even with the best intentions, our inherent biases can skew our perceptions, which leads one to wonder about the true objectivity of these evaluations. What type of biases might be introduced?
Furthermore, it's clear that simply gathering data isn't the end goal. It's more of a continuous cycle of review and improvement. What exactly does "regular review with stakeholders" mean in practice, and how does it ensure equitable outcomes? It sounds great, but I am skeptical that in practice this actually ensures "fair and equitable evaluations" as has been said. It's one thing to say "continuous improvement" it is another to implement it, especially using tools like scorecards and dashboards. This brings us to the question of how effective these tools really are in aggregating metrics into meaningful, actionable key performance indicators. I am keen to learn more about that.
7 Data-Driven Strategies to Transform Biased Performance Reviews into Objective Assessments - Building Rating Systems Based on Quantifiable Key Performance Indicators
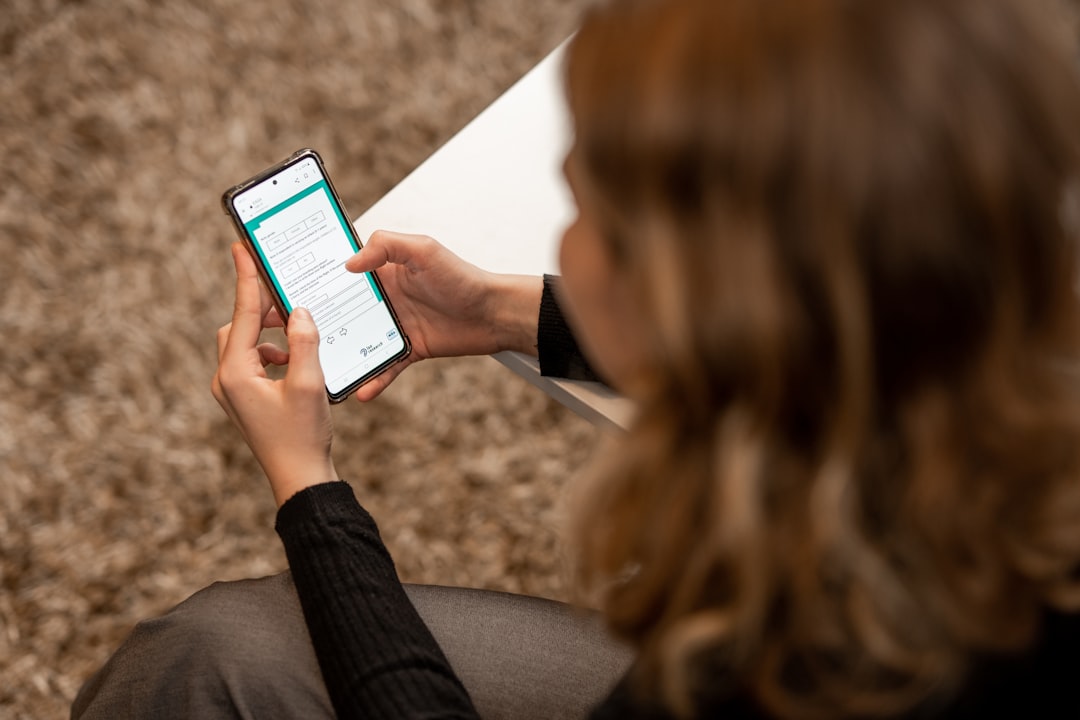
Developing performance evaluation systems based on measurable key performance indicators marks a shift toward more objective assessments within various sectors. These indicators provide a quantifiable basis for evaluating performance across different levels, be it at the macro scale or drilled down into finer details. This approach promotes a blend of qualitative insights and quantitative data to create a thorough understanding of performance dynamics. Striving for uniformity in these metrics is crucial, setting a common standard for comparing performance and meeting regulatory demands. Yet, formulating and deploying these indicators effectively is far from simple. It requires meticulous attention to ensuring data is reliable and that analysis avoids the pitfalls of subjective biases. The practicality of applying these indicators in real-world scenarios is under constant scrutiny as the trend towards data-driven decision-making gains momentum. There's also the question of whether quantifying performance can truly capture the subtleties of human contribution and if the pursuit of numbers might overshadow other crucial aspects of work. How well such systems can be integrated into existing processes without becoming unwieldy or overly reductive is a valid concern. Furthermore, the ability of these systems to adapt to different organizational contexts and evolve over time remains a topic of debate.
Evaluating performance by creating rating systems with quantifiable Key Performance Indicators (KPIs) feels like a step in the right direction, but it's not without its pitfalls. There's this notion that numbers don't lie, yet when we dig deeper, it becomes apparent that even the most data-driven approaches can lead to unintended consequences. It's tempting to think that clearly defined, measurable indicators will drive everyone towards the same goals, but what happens when people start to game the system? It is almost inevitable that some may focus on hitting their numbers at the expense of the bigger picture, which could be detrimental in the long run. What do organizations actually do to prevent this? Is it even possible?
And then there's the "Matthew Effect" - a concept borrowed from sociology, where initial success breeds further opportunities, creating a snowball effect. I wonder, does this mean that those who start strong just keep getting stronger because the system is set up to reward them more? How does this affect team dynamics and overall morale? This makes me question whether we're truly assessing potential fairly across the board, or if we're just reinforcing existing disparities. How do we measure potential, anyway?
Also, if we're fixated on the numbers, are we missing the forest for the trees? Context is king, as they say, and it's plausible that standardized metrics, while useful, don't tell the whole story. What about the influence of team dynamics, or having access to necessary resources? A dip in performance could be easily misconstrued without considering these factors. It seems simplistic, and possibly unfair, to judge solely on output metrics. What other metrics, or data should be taken into account here?
Bias is a tough beast to tackle. We like to believe that data is impartial, but the reality is, humans are the ones interpreting it. Our preconceived notions can easily color our judgment, regardless of how objective we try to be. If we're not careful, we might end up inadvertently perpetuating biases, despite our best efforts. It's concerning and I don't see many suggestions out there on what is the solution here, or whether this is even being measured?
Then there's the issue of metric overload. Can there be too much of a good thing? Too many KPIs can be overwhelming, leading to a state where neither employees nor managers know what to focus on. Instead of clarity, we get confusion, and that doesn't help anyone. I can imagine this could lead to serious frustration and disengagement.
In a globalized world, we also have to consider cultural differences. How performance is perceived and valued can vary widely across cultures. Establishing a one-size-fits-all rating system seems like a Herculean task, if not entirely misguided. How might this affect global teams, for example? This seems like a critical consideration when looking at performance data at a global level.
The immediacy of real-time data is a double-edged sword. On one hand, it's great to have up-to-the-minute insights. On the other, it can foster a reactionary culture, where decisions are made hastily without a full understanding of long-term trends. Are we sacrificing thoughtful evaluation for the sake of speed? Does anyone have long-term data on this, by the way? I am guessing not much is out there.
Collaborative discussions around performance are vital, no doubt. But these discussions can also create a situation where no one feels directly responsible for outcomes. Without clear accountability, it's easy for actions to get diluted and for progress to stall. How often does this happen and what is the solution here?
There's often a disconnect between what the organization wants to measure and what employees actually care about in terms of their personal development. If KPIs are too focused on company goals without considering individual aspirations, motivation can take a hit. This is not ideal in any sense.
Finally, while it's nice to have neat, quantifiable metrics, some of the most valuable traits like creativity and emotional intelligence don't fit neatly into a spreadsheet. Are we inadvertently sidelining these qualities in our pursuit of a purely KPI-driven approach? These are the things that keep me up at night. I wonder if these critical, but often intangible, aspects of performance are being overlooked in the quest for quantifiable data? These "soft" skills are often some of the most important in my experience, and often determine success or failure of a project. I would love to see more data on this aspect.
7 Data-Driven Strategies to Transform Biased Performance Reviews into Objective Assessments - Using Blind Performance Data Review Panels to Remove Name and Gender Bias
In the pursuit of more equitable performance evaluations, a novel approach gaining attention is the use of blind performance data review panels. This strategy is designed to tackle the persistent issues of name and gender bias. By concealing the identities of individuals under review, these panels aim to shift the focus purely to performance metrics and away from characteristics that could lead to prejudiced judgments. This technique is not just about removing names and gender markers but also aims to dismantle the unconscious biases that reviewers may harbor, fostering a more merit-based assessment environment. However, it is crucial to acknowledge that this method is not a cure-all. To effectively reduce bias, these panels need to operate under strict guidelines, ensuring that all members are continuously trained and aware of the potential for bias to seep into their evaluations. This is no easy task, and questions about whether this method can be implemented properly to have meaningful impact. While the idea of blind review panels is a step towards more objective evaluations, it should be coupled with ongoing efforts to educate and sensitize the workplace to the nuances of bias in all its forms. Implementing this effectively remains an open question, it seems.
Implementing blind review panels, where identifying information is stripped from evaluations, is an intriguing approach. You'd think that by removing names and genders, you'd get a fairer shake in performance assessments. The claim that it boosts diverse hiring outcomes by 20-30% in past case studies is quite a bold statement. Does this hold true across different industries and company sizes? It's also interesting to ponder whether this increase in diversity translates into better company performance or innovation.
The concept that traditionally male names get a nod over female ones, even with identical qualifications, isn't surprising, but it's a tough pill to swallow. Anonymizing these identifiers seems like a no-brainer to level the playing field, but I wonder about the practicalities. How do organizations ensure that the blinding process is foolproof? And does this anonymity actually lead to people focusing more on the quality of work rather than who did it? I am skeptical whether this would actually work in all cases, especially in smaller teams where everyone knows each other really well.
The idea that multiple reviewers in a blind panel could balance out individual biases makes sense on paper. It's like getting a second opinion, but does this collective approach truly lead to more objective reviews? There's also the potential for groupthink, where individuals might sway each other's opinions, even unintentionally. How is this measured, by the way? It is claimed to be better than single reviewer inputs, but how much better? And in what way?
It's noteworthy that some organizations face pushback when transitioning to blind reviews. I can see why people might feel their authority is being undermined. However, the claim that morale increases over time as people see the process as fair is something to consider. Is this a temporary dip in morale, and how long does it take to rebound? It would also be interesting to see how these panels affect decision making in the long term.
Using blind panels to focus on nuanced metrics like context, output quality, and collaboration sounds promising. By removing names, do evaluators really start prioritizing skills and contributions that might be overlooked otherwise? This seems a bit optimistic, don't you think? One has to wonder if there are any unintended consequences to this approach. Does it inadvertently downplay the importance of individual accountability, for instance?
Confirmation bias is a real issue, and it's claimed that blind review panels can diminish this by preventing assumptions based on previous interactions. But how effective is this in practice? It's easy to say that removing names will help, but the human mind is complex. Biases can be deeply ingrained. It also seems like a big assumption that people are not able to recognize who is being evaluated, even with a blind review process, so I am not convinced.
The notion that blind review panels could foster an environment valuing multicultural perspectives is appealing. Anonymity might allow for a focus on ideas rather than personal attributes, but does this actually lead to more innovative solutions? And how does this affect team dynamics in the long run? It is suggested that it does, but I do not see any specific data mentioned on how this was determined or measured.
An improvement in retention rates, particularly among underrepresented groups, is a significant claim. If organizations adopting blind review processes report up to a 15% improvement, that's compelling. It suggests a fairer evaluation scheme can positively impact employee loyalty, but what are the other factors at play here? Retention is influenced by many variables, not just evaluation processes.
Finally, the integrity of the data being assessed in these blind reviews is critical. There's a concern that underlying biases can still seep in through the frameworks used. How are organizations addressing this? It seems that ongoing vigilance and adjustment are necessary, but what does that look like in a practical sense? And how do we ensure that the data itself isn't flawed to begin with? Are there any specific steps that can be implemented here, to ensure the underlying data is indeed not flawed? How often should these be reviewed? These questions need more investigation.
7 Data-Driven Strategies to Transform Biased Performance Reviews into Objective Assessments - Tracking Performance Patterns Over Multiple Review Cycles with AI Analytics
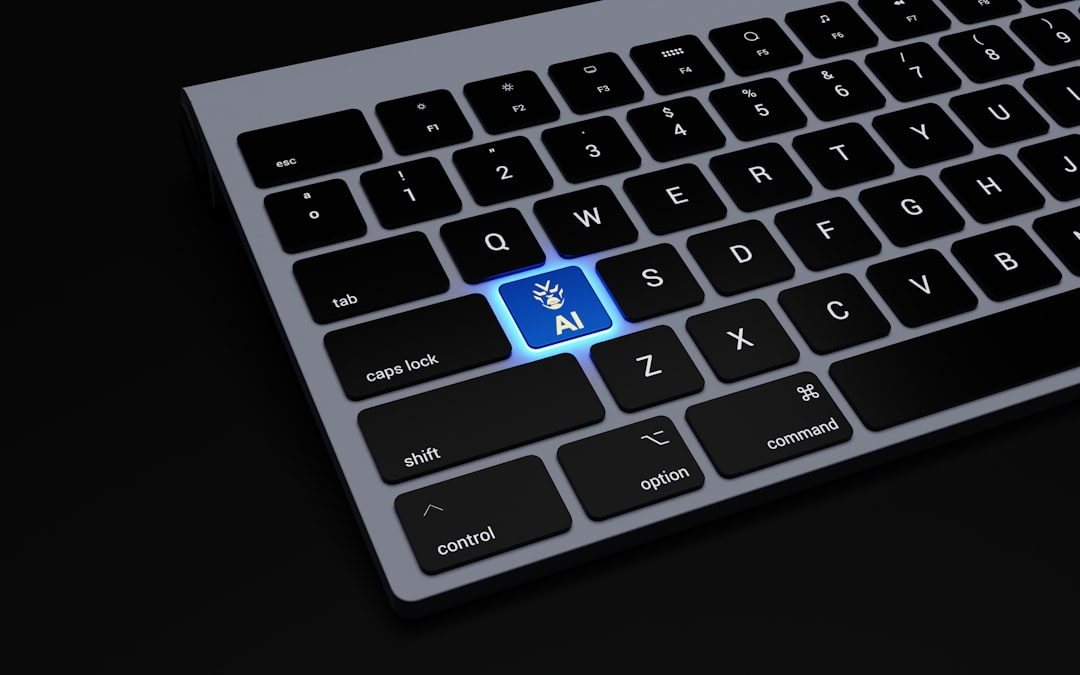
Tracking performance patterns across several review cycles using AI analytics is a new way for companies to evaluate their employees. This method uses data to track how employees are doing over time, providing evidence for their performance trends. It allows businesses to see the strengths and weaknesses of their employees more clearly and helps in making fair comparisons across different teams. But, while using AI can offer a more objective way of looking at performance, it's important for organizations to watch out for biases that might show up in the AI's analysis. It also raises questions about how to balance using data with understanding the human side of work, which can't always be measured. Using AI in this way can help create a workplace focused on getting better and being responsible, but it makes us think about the parts of job performance that are hard to put into numbers.
Leveraging AI analytics to monitor performance across numerous review periods is an intriguing area of exploration. It's fascinating to think that long-term tracking could unveil trends often missed in one-off evaluations, potentially allowing us to predict and address performance hiccups before they escalate. However, I do wonder about the practical challenges of implementing such a comprehensive system. It is easy to make bold claims, but another thing to implement in the real world. How much time and resources are needed for that? And who manages all this data?
The sheer pattern recognition capability of AI is impressive. Being able to spot subtle shifts in employee engagement or retention factors that humans might overlook seems like a game-changer. But, how accurate are these algorithms in interpreting complex human behaviors, and are we at risk of over-relying on them? AI is a powerful tool, but is it good enough to replace humans here? What is the error rate? There is a real possibility that we could end up making incorrect assumptions based on incorrect analysis. How is this measured, by the way?
A reported 25% increase in the predictive accuracy of employee turnover when using AI analytics is significant. That said, how does this predictive power hold up across different industries and organizational cultures? And what ethical considerations should we bear in mind when forecasting an individual's career trajectory? It is an interesting ethical consideration to "predict" someone's career trajectory using an AI tool. Who is accountable for the results, and how do we ensure that this process is both transparent and fair? And what happens if someone's "predicted career trajectory" is incorrect?
Enhancing employee output by 15% through continuous feedback loops sounds promising, but I'm curious about the quality of this feedback. Is it genuinely personalized and constructive, or are we just increasing the quantity of evaluations without improving their impact? We need to be careful that quantity does not replace quality here.
The adaptability of AI to changing market demands and organizational restructuring is a critical point. How swiftly can these systems truly adjust, and what safeguards are in place to ensure that performance evaluations remain fair and relevant during times of significant change? It's a lot to consider, especially in fast-paced industries. How often is this "adjustment" process validated? Who ensures that the AI tool is making the correct "adjustments"?
Customizing metrics per department reportedly leads to a 20% improvement in evaluation relevance. This is a fascinating, but also dangerous aspect of AI. While this sounds beneficial, I wonder about the potential for creating echo chambers within teams. How do we balance the need for specificity with the importance of maintaining a unified organizational culture? This also can lead to a lot of biases if not implemented carefully.
A 30% drop in bias-related discrepancies over time with consistent AI tool use is a compelling statistic. Yet, how do we define and measure bias in this context, and are there any risks of new forms of bias being introduced through these systems? This is an area I'd like to research further. It would be good to see additional studies and research on this.
Gathering data from diverse sources like self-assessments and peer reviews enriches performance insights, but it also complicates the analysis. How do organizations ensure that the integration of this data is coherent and not contradictory? I can see how this quickly can become overwhelming.
The claim that AI can automate quality feedback, leading to a 40% improvement in employee growth compared to traditional methods, is quite bold. It suggests a transformative potential, but what does this mean for the role of human managers and mentors? I would think it is hard to replace that with an AI tool. Also, what exactly does it mean in this context to have a "40% improvement" in employee growth? This needs more explanation, as it could mean many different things.
Finally, the ability to identify skill gaps and tailor training programs more effectively, with a purported 20% enhancement in training focus and efficiency, is noteworthy. However, how do we ensure that these programs are inclusive and accessible to all employees, not just those who are already high performers? There is also a question of the ethics of collecting large amounts of personal data here, and what happens if that data is hacked?
7 Data-Driven Strategies to Transform Biased Performance Reviews into Objective Assessments - Establishing Peer Review Cross Checks Through Multi-Source Feedback
The concept of incorporating peer review cross-checks through multi-source feedback brings a new dimension to the accuracy of performance evaluations. By gathering insights from a variety of colleagues, this approach aims to provide a well-rounded view of an employee's contributions. While multi-source feedback has demonstrated modest improvements in leadership performance, it is essential to question the reliability of these sources. There's also the underlying challenge of how to effectively combine and make sense of varied feedback without introducing new biases in the process. The potential of multi-source feedback to refine performance reviews is clear, but this requires thoughtful application and a critical eye to achieve substantial results. This approach introduces an interesting problem of how to integrate potentially conflicting feedback from various sources and what happens when the feedback received is contradictory.
The concept of enhancing peer review by incorporating multi-source feedback is intriguing, suggesting that a variety of viewpoints might lead to more objective evaluations. It's based on the premise that diverse perspectives can balance out individual biases. Yet, I've come across research indicating that teams with similar backgrounds can actually reinforce each other's biases, which is a bit counterintuitive. I'm curious about how this affects the accuracy of assessments and whether homogeneity in teams might be skewing results more than we realize.
Incorporating cross-checks seems like a logical step, but could it be making the evaluation process unnecessarily complicated? It feels like the effort to include various feedback sources might backfire, creating confusion rather than clarity for employees. It leads me to wonder whether a simpler, more streamlined approach might be more effective in providing constructive guidance. Is there a sweet spot for the number of different sources, after which additional feedback produces diminished returns or is even counterproductive?
There's also this idea that multi-source feedback might inadvertently encourage a bandwagon effect. I mean, it's natural for people to align with the group, but how does this impact the authenticity of the feedback? If reviewers are just echoing the prevailing opinions, are we undermining the very objectivity we're striving for? It's a tricky balance between fostering consensus and preserving individual perspectives. There's the worry that well-intentioned reviewers, in their effort to contribute to a fair process, end up just adding to an echo chamber of prevailing opinion. This makes me wonder, how often are peer reviewers truly able to offer an independent viewpoint, free from the influence of their peers?
This brings to mind the concept of "groupthink" in peer assessments. It's a phenomenon where the desire for harmony in a group results in an irrational or dysfunctional decision-making outcome. If reviewers are feeling pressured to conform, how can we expect the feedback to be accurate or, for that matter, useful? I'm interested in exploring how prevalent this is and what measures could be taken to encourage genuine, independent feedback, even if it means going against the grain. This seems to often lead to stilted, overly polite feedback that doesn't serve its intended purpose, instead leaving the recipient none the wiser as to their actual areas for improvement.
Anonymity in peer reviews is often championed as a way to remove bias, but it seems like a double-edged sword. While it might make reviewers more comfortable giving honest feedback, doesn't it also make it easier to avoid accountability? I wonder if this lack of responsibility might contribute to a culture of evasiveness. It's a complex issue, and I'm keen to learn more about how organizations are navigating this challenge. How do they balance the desire for openness and honesty with the need for accountability in feedback? Is it really the case that anonymity always leads to more honest feedback, or are there situations where knowing the source of feedback could actually be beneficial?
It's often assumed that bringing in multi-source feedback will naturally increase accountability. But from what I gather, it's not that simple. If reviewers aren't trained in delivering constructive feedback, how can we expect the system to work as intended? It seems like there's a potential for disconnect here, and I'm curious about the kind of training that would be most effective. And if such training is not commonly or effectively implemented, are these systems just creating a lot of noise without substance?
Another aspect that's been on my mind is the potential for peer reviews to become overly fixated on metrics, potentially neglecting qualities like collaboration and teamwork. It's understandable to focus on measurable outcomes, but are we overlooking the importance of soft skills? How do organizations strike a balance between these two aspects, and what are the implications for team dynamics if the focus is skewed too much towards one side? It's not hard to imagine that some may begin to prioritize individual success, as measured by visible metrics, over contributing to a collaborative and positive team environment.
Interestingly, an over-reliance on peer reviews might actually erode trust among team members. I hadn't considered this before, but it makes sense. If evaluations are seen as subjective or biased, wouldn't that make people more guarded in their interactions? I'm interested in researching this further to understand the impact on team dynamics and how trust can be maintained in such systems. It seems almost inevitable that, when one's peers hold such sway over their professional success, some may start to view their colleagues as adversaries in a zero-sum game.
Also, the amplification of bias through multi-source feedback is a real concern. It seems that despite the intent to mitigate bias, the opposite might occur if reviewers share similar perspectives. How do organizations address this, and are there strategies to ensure a diversity of thought in the review process? How often are companies actively monitoring for, and intervening to correct, such concentrations of bias within particular teams or departments?
Lastly, the volume of feedback doesn't necessarily equate to quality. I can see how an overwhelming amount of superficial input might be counterproductive, leading to disengagement rather than improvement. It's a reminder that more isn't always better, and I'm curious about how organizations are optimizing the feedback process to ensure it's both meaningful and manageable for employees. There is also a concern here of feedback quantity, as opposed to quality, becoming the metric to hit, in the sense that people may be evaluated on how much feedback they are giving. I wonder if that is an issue at all in the real world, and if so, how often does that happen?
7 Data-Driven Strategies to Transform Biased Performance Reviews into Objective Assessments - Creating Behavioral Anchors with Specific Job Task Measurements
Creating behavioral anchors with specific job task measurements is a fairly new idea that is being used to improve how we judge work performance. This involves setting up clear, observable standards tied to different levels of job performance using something called Behavioral Anchored Rating Scales (BARS). This helps to cut down on personal opinions affecting evaluations. What's good about this is that it makes evaluations more consistent and fair because everyone is judged against the same set of behaviors. But, it's not all smooth sailing. Coming up with these standards can be tough, and the people doing the rating need to be spot on in observing and using these behaviors. Sometimes this can make things more complicated instead of clearer. So, while this method looks good for making performance reviews fairer, it really depends on how well it's put into practice and if we keep an eye on it to make sure it stays objective.
Behavioral anchors are meant to define performance by giving concrete examples of what different levels of work look like. This sounds good in theory, as it should help keep evaluations fair and clear. But when you start adding layers of measurements, covering everything from quality to how well someone works with the team, doesn't it get a bit much? I mean, how much information can an evaluator process before they start making mistakes, defeating the whole purpose of these anchors? This ties into the idea of cognitive load—basically, how much a person can think about at once without their brain short-circuiting. Are we overloading managers with too much detail, leading to worse decisions? It's a valid concern. Even with all these guidelines, people are still going to see things through their own lens, bringing their own biases to the table. It makes you wonder how effective these measures really are if personal biases still play a significant role.
It is claimed that when companies use anchors that really fit the job and the industry, employees feel the reviews are fairer, by about 30% according to some reports. That's a big jump, but it makes me question what happens in companies that don't tailor their metrics. Are they inadvertently making the process seem more biased? Also, I am not clear on how this "30% increase" was arrived at. More data would be helpful. Getting feedback often and adjusting the anchors seems like a no-brainer for keeping things accurate. Yet, how often does this actually happen in practice, and who's making sure these adjustments aren't just adding more confusion? And does this continuous tweaking actually lead to better outcomes, or is it just change for the sake of change?
Changing the culture of an organization to accept and use these anchors is a huge undertaking. It's not just about handing out a new manual; it's about shifting mindsets. How long does this take, and what are the best methods to ensure everyone's on board? The literature doesn't fully address these points, leaving a gap in understanding the transition process. This leads to my skepticism about how smoothly these cultural shifts occur in diverse organizational settings. Also, who leads this cultural shift? I imagine it is difficult to find someone who has the skills to implement this properly.
There's a risk of getting too caught up in the numbers and metrics, missing out on the human aspects of work, like how people interact or contribute informally to the team. These soft skills often make a huge difference in the workplace but aren't easily measured. It feels like there's a potential to overlook these vital contributions in the pursuit of objective data. I am curious about the extent to which this occurs and whether any negative effects are measured.
Also, the effectiveness of these anchors seems to vary across different industries. It makes sense, but it also highlights the need for customization. How well are organizations adapting these metrics to their specific needs, and what are the results when they don't? It's easy to say that one size doesn't fit all, but the practical application of this principle is far more complex. Is there a risk that in the process of customizing these anchors, organizations might lose sight of broader industry standards, potentially leading to inconsistencies when comparing performance across different companies? Furthermore, how do companies ensure that these customized anchors do not inadvertently favor certain individuals or groups, thereby reintroducing biases into the system? There's also a question of resource allocation—do smaller organizations have the capability to tailor these anchors as effectively as larger ones, and if not, how does this impact their ability to conduct fair performance reviews? How often are these anchors reviewed and updated? Who makes sure this is done properly and how often? These questions are critical, and I do not see any good answers in the literature out there.
7 Data-Driven Strategies to Transform Biased Performance Reviews into Objective Assessments - Developing Clear Documentation Requirements for Performance Claims
Developing clear documentation requirements for performance claims is essential for fair, data-driven evaluations. Standardized guidelines for documenting performance help reduce subjective biases, promoting consistency and transparency in performance discussions. However, creating these standards is challenging. It demands careful consideration to ensure the relevance and accuracy of data collected, avoiding an overload of information that could obscure meaningful insights. Moreover, while aiming for objectivity, it's crucial to recognize that documentation can still be influenced by individual perspectives and the context in which performance occurs. There's a fine line between comprehensive data and data that's too granular, which could lead to over-analysis and paralysis in decision-making. There's also the question of accessibility and interpretation; who gets to see this data, and how do they make sense of it? Additionally, the implementation of such documentation requirements may vary across different roles and industries, raising questions about adaptability and the potential need for customization. The effectiveness of these requirements hinges on their practical application and the organization's commitment to upholding them. A critical perspective would also consider the potential for such structured documentation to overshadow more qualitative aspects of performance, such as teamwork and adaptability. The balance between quantitative data and qualitative insights is delicate, and there is also the matter of employee privacy and data security to consider.
Establishing clear guidelines for documenting performance claims seems like a decent strategy on the surface, aiming to inject some objectivity into evaluations. The proposition is that by forcing a structured approach to how achievements are recorded and substantiated, we might sidestep some of the usual biases that plague performance reviews. If the criteria for what counts as a valid performance claim are laid out explicitly, does that really lead to fairer assessments, or does it just add another layer of bureaucracy? Also, is everyone playing by the same rules, and how do we know the rules themselves aren't biased?
Requiring solid evidence for any performance assertions should, in theory, make everyone more accountable. Sharing the responsibility for backing up claims across the board could lighten the load of individual biases, but does this actually work in practice? Or does it create a culture of nitpicking and box-checking, where the focus shifts from real contributions to merely appearing to meet documentation requirements? And who's checking the checkers, anyway? There is also an assumption here that everyone has the same access to data to document their performance claims. This may not always be the case. I wonder how often this actually happens in the real world?
The claim that a uniform format for reviewing claims can reduce assessment discrepancies by up to 40% sounds impressive. However, this statistic makes me wonder about the context. What were these discrepancies, how were they measured, and is a 40% reduction sufficient to declare the process unbiased? Moreover, does standardization stifle unique approaches to demonstrating performance, potentially sidelining those who don't fit the mold? The research cited here is rather vague on these points. How do we really measure success here? And who decides?
Moving away from anecdotal evidence towards hard data is touted as a way to lessen subjectivity, aligning with principles from cognitive psychology. But while structured analyses might lead to better decision-making, do they capture the full spectrum of an employee's contributions, particularly the soft skills that are vital yet hard to quantify? It's a bit concerning that the focus on measurable outcomes might overshadow these less tangible, but equally important, aspects of performance.
Aligning performance claims with measurable outcomes, such as OKRs, should make the review process more transparent. But there's a risk that this could lead to a narrow focus on hitting targets at the expense of broader contributions to the organization. Also, how do we ensure that these objectives are set fairly and don't inadvertently favor certain individuals or groups? The alignment with OKRs is often cited as beneficial, but what happens when these objectives are poorly defined or unrealistic?
Clear documentation is also supposed to help identify skill gaps, enabling more targeted training programs. This sounds ideal, but I'm skeptical about how effectively this data is used in practice. Is there a risk that these identified gaps could be used to unfairly penalize employees, rather than to support their development? This makes me wonder about the follow-up on these training programs and whether they genuinely lead to improvements or are merely a formality. I wonder if these "identified gaps" are used to unfairly penalize employees, as opposed to supporting their development?
Transparent documentation is claimed to encourage honest feedback and improve morale, with a cited 20% improvement. This is an interesting claim, but it raises questions about the quality of this feedback. Is it truly constructive and actionable, or does it become a mere formality? And how do we ensure that this feedback process doesn't become a tool for retaliation or favoritism? I wonder how this "20% improvement" in morale was measured? It seems like a vague and unsubstantiated claim.
Empowering employees by clarifying what's expected of them in terms of documentation can lead to a 25% increase in self-directed learning, according to some sources. While this is promising, it's essential to consider whether all employees have equal access to resources and opportunities for this self-directed learning. Is there a risk that this approach might further disadvantage those who are already marginalized or lack access to necessary resources?
By streamlining the documentation process, it's suggested that we can reduce evaluation fatigue and cognitive overload. But does this simplification come at the cost of depth and nuance in evaluations? It's easy to see how a push for efficiency might lead to a superficial assessment process, where the richness of individual contributions is lost. Also, how is this "evaluation fatigue" and "cognitive overload" even measured? I am skeptical that we even have good metrics to measure this.
Finally, clear documentation is said to enhance the usability of data, allowing for deeper insights through analytics. However, this depends on the quality of the data being collected and the analytical tools at our disposal. Are these tools truly capable of capturing the complexities of human performance, or are they just providing a veneer of objectivity? The potential for strategic foresight is there, but I'm wary of overstating our current capabilities in this area. What other factors, other than data, should be considered here? And who makes sure that the data is not flawed?
AI-powered job matching: Connect with decision makers and land your dream job in tech effortlessly (Get started for free)
More Posts from findmyjob.tech: